AI could ‘resolve GST classification burden’, says ATO assistant commissioner
Machine learning could help address the confusion and disruption stemming from the current GST classification system, according to an ATO assistant commissioner.
Speaking at a recent Tax Institute conference, ATO assistant commissioner, Tax Counsel Network, Gordon Brysland said food classification had become a “disruptive irritant” in the GST system leading to increased costs for both suppliers and the community.
While the introduction of the GST system was originally intended to simplify the complex classification rules associated with the previous sales tax system, this has not played out in practice, he said speaking at the Tax Institute National GST Conference
Mr Brysland said a multitude of arbitrary and confusing classification rules within the GST rules means suppliers and tax professionals have been left to deal with a significant compliance burden in this area.
He referred to the recent Federal Court decision in Simplot v Commissioner of Taxation [2023] FCA 1115 which examined whether certain frozen food products supplied and imported by food manufacturer Simplot Australia were classified as a ‘prepared meal’ and therefore subject to GST.
In her decision, Justice Hespe questioned whether the arbitrary rules contained within the current GST laws were a satisfactory basis for determining taxation liabilities.
Mr Brysland said Justice Hespe’s comments follow similar commentary from previous judges over a long period that has called out the dysfunctionality of food exemptions in both the sales tax and GST regimes.
While a simple fix to addressing the current classification issues would be to expand the GST base and reduce the exemptions in the current laws, Mr Brysland said this would be difficult in practice given the current political climate and a cost of living crisis.
The ability of the government to expand the GST base is also hampered by Peter Costello’s lock mechanism which requires the government to obtain the unanimous consent of beneficiary jurisdictions before making changes, he added.
However, Mr Brysland said artificial intelligence could play a substantial role in reducing the compliance burden with classification in this area.
“Given that base expansion is unlikely in the extreme and that the position of the courts is confirmed, the problems of classification practice all but demand that we actively consider if machine learning tools might be of some utility in reducing compliance burdens,” Mr Brysland said in a paper released alongside his presentation.
Mr Brysland said the University of Toronto is already exploring how machine learning could be used to address closed-end legal classification problems.
“Professor Ben Alarie and colleagues at the University of Toronto are at the frontier of machine learning research in the tax classification and prediction spheres,” he said.
“Out of the University of Toronto work came Blue J Legal, which operates as an international start-up and has partnered with hundreds of organisations. It has developed a wide range of tax classifier tools on the back of enhanced algorithms. These are used across the Canadian tax system by advisers, academics, government and, most significantly, by the Canadian Revenue Authority.”
The project has also produced a range of other classifiers, including for residency claims.
“The project also takes a US tax appeal every month and seeks algorithmically to predict the outcome. So far the success rate is 100 per cent,” he said.
Mr Brysland stressed that the use of algorithmic classifiers would need to be transparent and there would need to be accountability at a human level for decisions that are made.
“A problem with algorithms in general is that the basis on which they reach conclusions is often unknowable in practice – the so-called ‘black box’ problem,” he said.
“In answer to this problem, much work is being done on the ‘opening’ of black boxes. Legislation in some places (including Canada) requires algorithmic impact assessments. The new disciplines of ‘algorithm accountability’ and ‘technological due process’ have emerged.
Research firm DARPA is exploring ‘explainable artificial intelligence’ aimed at creating systems that explain both their decisions and those of other systems. The strategy is to recruit simpler models to unlock the secrets of more complex ones, said Mr Brysland.
“One example, called ‘local interpretable model-agnostic explanations’ (LIME), seeks to isolate those factors which were most likely determinative in an algorithmic classification decision,” he stated.
The ATO has already developed an Automation and Artificial Intelligence Strategy to deliver the new technologies across the organisation.
“AI is used as a tool to assist the work of the ATO, but decision-making always remains in the hands of humans. The ATO holds itself accountable to six data ethics principles when collecting, managing, sharing and using data,” said Mr Brysland.
“The ATO uses machine learning models and natural language processing to identify unpaid liabilities and prevent tax fraud. Jeremy Hirschhorn has stated – ‘Importantly, leveraging data and analytics does not replace the human element: rather it frees up our people to focus on tasks requiring human judgment and empathy’”.
About the author
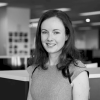